My research interests are in artificial intelligence at the intersection of computer vision and computer graphics. I am interested in 3D scene understanding and representation learning. I am advised by Charless Fowlkes.
Education
Since 2017, Ph.D. in Computer Science, University of California, Irvine (advisor: Charless Fowlkes)
2017, M.S. in Computer Science, University of Illinois at Urbana-Champaign (advisor: Derek Hoiem)
2015, B.S. in Computer Science, University of Illinois at Urbana-Champaign
Publications
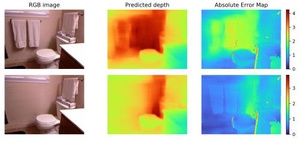
Domain Decluttering: Simplifying Images to
Mitigate Synthetic-Real Domain Shift and Improve Depth Estimation
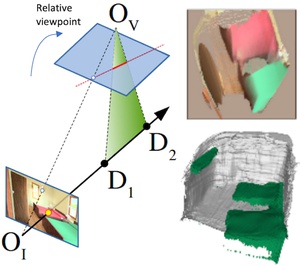
3D Scene Reconstruction with Multi-layer Depth and Epipolar Transformers
- / Paper
- / Poster
- / Project Page
- / Slides
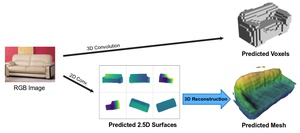
Pixels, voxels, and views:
A study of shape representations for single view 3D object shape prediction
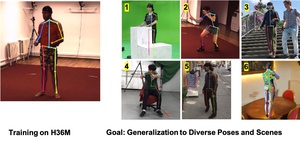
Predicting Camera Viewpoint Improves Cross-dataset Generalization
for 3D Human Pose Estimation
- / Paper
- / Project Page
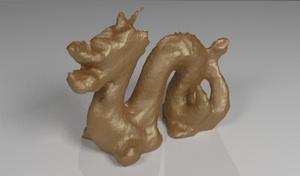
3DFS: Deformable Dense Depth Fusion and Segmentation for
Object Reconstruction from a Handheld Camera
Selected Projects
- Learning viewer-centered projections for 3D shape completion (2017) [Overview] [Thesis]
- tf.nn.conv3d_transpose python op in tensorflow:master (2016) [GitHub pull request]
Internships
Google
Research Intern
Irvine, CA
June 2021 - Sept 2021
Snap Inc.
Research Intern
Santa Monica, CA
June 2019 - Sept 2019
Google
Software Engineering Intern
New York, NY
May 2016 - Aug 2016
Google
Software Engineering Intern
Pittsburgh, PA
May 2015 - Aug 2015
Amazon Web Services
Software Engineering Intern
Palo Alto, CA
May 2014 - Aug 2014
Amazon.com
Software Engineering Intern
Seattle, WA
May 2013 - Aug 2013
Talks
- Berkeley Artificial Intelligence Research (BAIR) Lab, 3D Scene Reconstruction with Multi-layer Depth and Epipolar Transformers. CA, USA. Sept. 17, 2019. [slides]
- Stanford Vision and Learning Lab (SVL) Group Meeting, 3D Scene Reconstruction with Multi-layer Depth and Epipolar Transformers. CA, USA. Sept. 16, 2019. [slides]
- KAIST Scalable Graphics, Vision, & Robotics (SGVR) Lab, 3D Scene Reconstruction with Multi-layer Depth and Epipolar Transformers. Daejeon, Korea. Oct. 2019. [slides]
- UCI AI/ML Seminar Series, Multi-layer Depth and Epipolar Feature Transformers for 3D Scene Reconstruction. CA, USA. Apr. 15, 2019. [page]
Professional Services
Conference Reviewer
ICLR 2021, ECCV 2020, NeurIPS 2020, CVPR 2020, ACCV 2020, ICLR 2020, AAAI 2020, ICCV 2019, CVPR 2019, WACV 2020, BMVC 2019, ACCV 2018, WACV 2019
Journal Reviewer
T-PAMI, IEEE Access
Research Topics
- Viewer-centered 3D reconstruction.
- Geometric output representations.
- 3D scene understanding.
- Learning novel objects.
- Multi-task learning.
- Learning from synthetic images.
- Adaptive computation.